STGL: Self-Supervised Spatio-Temporal Graph Learning for Traffic Forecasting
DOI:
https://doi.org/10.70891/JAIR.2025.040001Keywords:
spatio-temporal graph, self-supervised learning, traffic forecasting, contrastive learningAbstract
As urbanization intensification, traffic forecasting emerges as a critical challenge due to the complex spatio-temporal dependencies and data scarcity in traffic networks. Although spatio-temporal graph neural networks (STGNNS) have demonstrated certain efficacy, these methods cannot effectively model the complex characteristics of traffic data. Meanwhile, their performance is also constrained by the limited data volume and the scarcity of labels. To solve the aforementioned issues, we propose STGL, a self-supervised spatio-temporal graph learning framework for traffic forecasting. STGL utilizes a dual-module architecture to effectively model complex spatio-temporal dependencies in traffic data. Specifically, it integrates a dynamic graph convolution module to capture evolving spatial dependencies, and it utilizes a temporal convolution module leveraging dilated causal convolutions and gated mechanisms to model long-range temporal dependencies. To further enhance representation learning, STGL incorporates a contrastive learning with sample generation and negative filtering. By combining these components, STGL provides a robust solution for traffic forecasting under data-short conditions. Extensive experiments are conducted on PEMS04 and PEMS08, which shows the superiority of STCL.
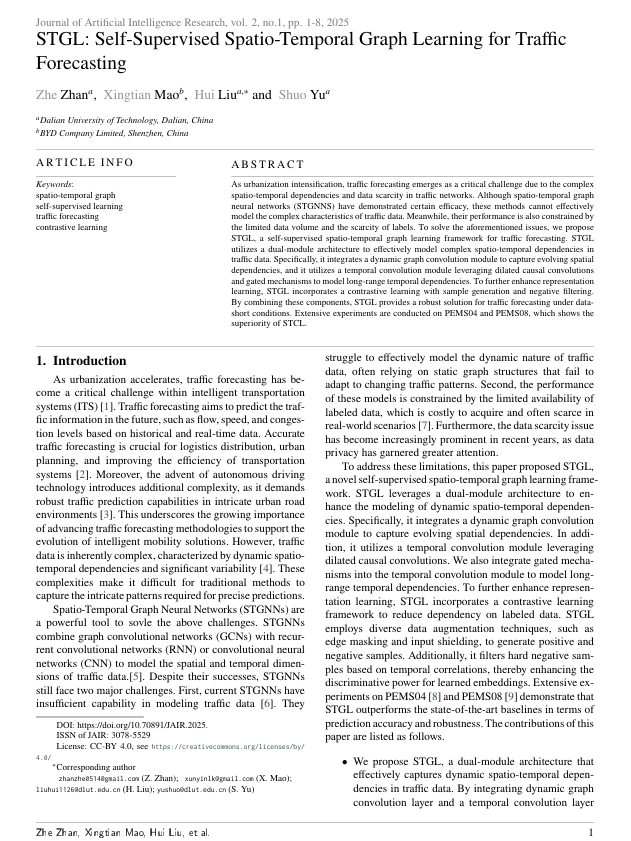